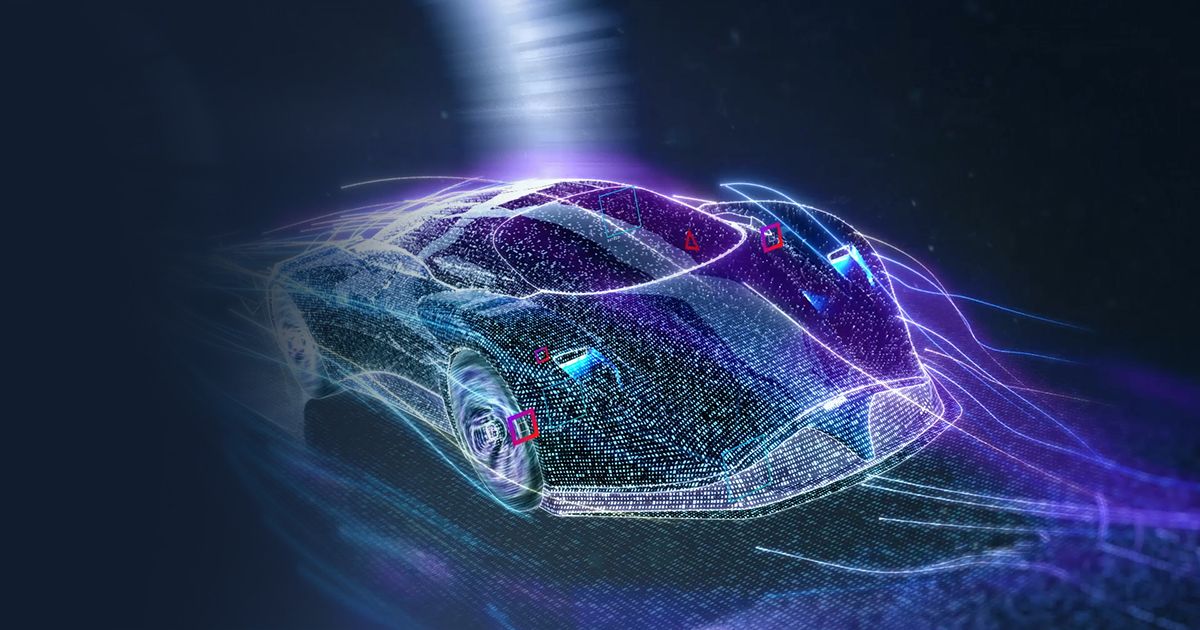
Ensuring Safety in Autonomous Vehicles: Challenges and Innovations
In recent years, there has been a significant growth in research related to the development of autonomous vehicles (AV) due to the increasing interest of car manufacturers and companies that provide transportation services for a high level of vehicle automation.
In a talk with a software engineer from the company dSPACE engineering, we learned more about the complexity of creating an AV and what it takes to achieve, maybe the most important element, safety, for the driver and all the external factors.
How to achieve safety?
A prerequisite for ensuring the reliability and safety of such autonomous systems is their comprehensive testing. The reason for this is that inadequate or ineffective testing could omit the detection of potential defects and malfunctions in the system, and consequently lead to serious accidents in road traffic.
One way to ensure the safety of an AV is to drive it "enough" on real roads. How far an AV must travel to adequately assess its safety and reliability is still debated. Some studies claim that hundreds of millions of kilometers of driving are needed to statistically compare AV safety versus human driver performance. However, this would take decades and is impractical and uneconomical. This is because regular road traffic, which occurs most of the time, is usually non-critical.
Therefore, the focus of AV testing has shifted from a distance-traveled approach to a scenario-based approach.
Scenario-based testing is usually performed in simulation with Software-in-the-Loop (SIL), but can also be performed with Hardware-in-the-Loop (HIL) or in the real world, such as on proving ground (also known as test tracks) or in regular road traffic.
Despite the incredible benefits of using a scenario-based approach to test AVs, identifying the relevant test scenarios, especially the critical ones (so-called "edge" scenarios), presents a certain challenge. This is because there is still no universal agreement on what exactly constitutes a critical scenario, although the various interpretations in the literature share a great deal of similarity.
For example, some authors describe a critical scenario as a dangerous road situation that can lead to an unsafe decision for an AV and appropriate countermeasures must be taken immediately to avoid a collision. Therefore, the success of a scenario-based testing approach largely depends on the ability to find critical scenarios.
What when it comes to the complexity of human behavior?
In addition to the above, significant efforts are being made to improve road traffic safety to vulnerable road users such as pedestrians, who participate in a significant proportion of fatal traffic accidents. Therefore, various safety systems are integrated into AVs aimed at carrying out emergency interventions in critical situations typical of urban environments, for example, automatic braking or sudden turning of the vehicle, to completely avoid or at least mitigate a collision. One of the main obstacles to the wider adoption of these systems is their sensitivity to false positive detections, in which action could be triggered unnecessarily. The timely activation of safety systems is also significant, where early action could irritate the AV user, and too late action could cause an unavoidable accident.
In the case of pedestrians, modeling is a challenging task, because it needs to capture the complex behavior of both pedestrians and drivers, as well as their interactions. The reason for this is the inherent complexity of human behavior, the influence of the environment, and numerous factors that determine pedestrians (for example, group size, age, gender, attention, trajectory, and the like).
As part of the research in which I participated at the Zagreb FSB, a pedestrian behavior model based on game theory was considered and further improved, which includes sub-models for the perception of the environment (estimation of the position and speed of the vehicle), expectations of the driver's intention (probability of stopping the vehicle), making decision (crossing the road or giving way to a vehicle), and ultimately the movement of pedestrians.
Game theory fits naturally in this context since vehicles and pedestrians can be thought of as adversarial agents competing for a crosswalk as a shared resource. For the proposed pedestrian behavior model to reflect reality as much as possible, it was calibrated on real vehicle-pedestrian interaction data.
The further direction of development implies the use of advanced machine learning methods, especially those related to stochastic modeling of a large number of pedestrians and vehicles, which behave intelligently and autonomously. The final step is to include such autonomous agents in simulation environments within existing dSPACE tools.