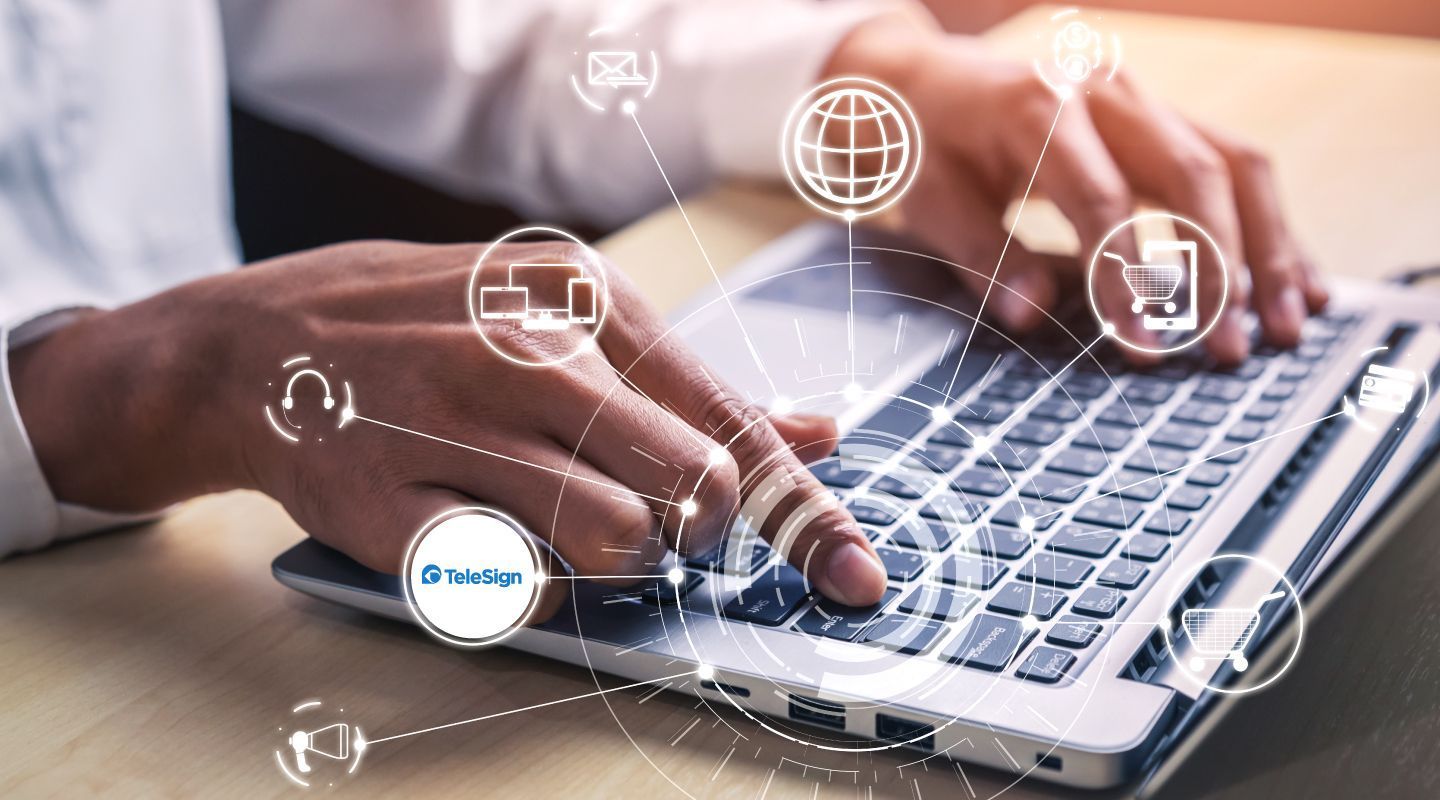
From Data Science to Business - Per aspera ad astra
Although a representative of the Data Science population may already have a complaint about the "direction" of the title, with the objection that the true counterpart of "from thorns to stars" would be "from business to Data Science", I would say that this is one of the prejudices, which The Data Science community needs to be freed up to have an easier time in business. In contrast to science, where the goal of Data Science is an approximation with mathematical (or any adequate) models, in business Data Science, is a form of monetization of science, i.e. we need to understand that the goal is inverse - we need to turn the mathematical model into a profitable reality.
The goal of this text is to consider some aspects, which can be useful guidelines in achieving that goal.
Data Science - the sexiest job in the 21st century
Although I am not personally a fan of this label, I will use it to summarize how naive the label can lead us in the wrong direction. Against this attractive label, we often come across a not-so-attractive reality. Business, without elementary knowledge of Data Science, most often lives in short-term clouds of "stardust" (where I do not mean the necessary cloud technologies 😊). On the other hand, Data Science personnel, who did not come from mathematics and related sciences, and who have no initiative to understand business, are reduced to hypothetical stories stuffed with complex terminology. The reality is that this kind of business and Data Science form a critical mass for the development of the company's mistrust of Data Science, instead of the development of Data Science in the company.
Companies that have seriously experienced Data Science transformation should not be recognized in any of the categories mentioned above. The question is how to reach that level of transformation...Data Science is considered the most popular profession today, but the bottom line is that a large number of organizations fail to successfully implement Data Science practices into their business and/or maximize the benefits of Data Science. The main reason for this is a fundamental misunderstanding and as a consequence the inability to provide the sound foundations of a Data Science organization, namely quality data and professional staff, not necessarily in that order. There is no correct answer to the question of whether for a successful data-driven business in companies, there should be "data already existing or Data Scientist". The only correct answer is that both are necessary for success, and the business needs to be able to intelligently orchestrate the organization and growth of the data and the team working with the data.
What defines data quality?
When we talk about data quality, there are two branches that stem from this node:
- data organization,
2. informativeness of data.
Although data organization is not necessarily the first step in introducing Data Science practices into the organization, it is certainly crucial if we want to achieve a serious business result. If the company is not able to initially assess the quality and quantity of the data it has, as well as those that would be useful to have for solving business problems, it is quite appropriate for the data organization to be temporarily in an ad-hoc manner. From the moment when the starting value of internal data, as well as the need for new data, and the scope and method of using it is determined, the organization of data in the form of an advanced data platform must become one of the top priorities of a data-driven company.
What a serious data platform should think about:
1.How to dynamically structure, organize, standardize and store data?
2. How to make stored data comfortable for further manipulation and transformation?
3. How to effectively deliver the result of the Data Science process to the user?
4. How to guarantee the security of confidential data?
The volume of data, the complexity of data transformations, and regulatory standards on data protection should be seen as the most important variables in the process of choosing a technology and architecture, which will fulfill the above-mentioned data platform needs.
The informativeness of the data, and the possibility to separate the signal from the noise, is what makes Data Science so "magical", and what can differentiate the company in the market. Validation of internal and/or finding external sources of data, which through the application of Data Science methods will contribute to the description/modeling of a real business problem, is a process that can begin even before having an advanced data organization. Moreover, the result of this step represents another important input for defining the final organization of the data. This is the segment that most serious data-driven companies mark as real data quality because it represents the moment when research begins to acquire a business dimension. Nevertheless, we should not forget that the overall quality of data, which is necessary for a long-term data strategy, is a synergy of healthy form and informative context.
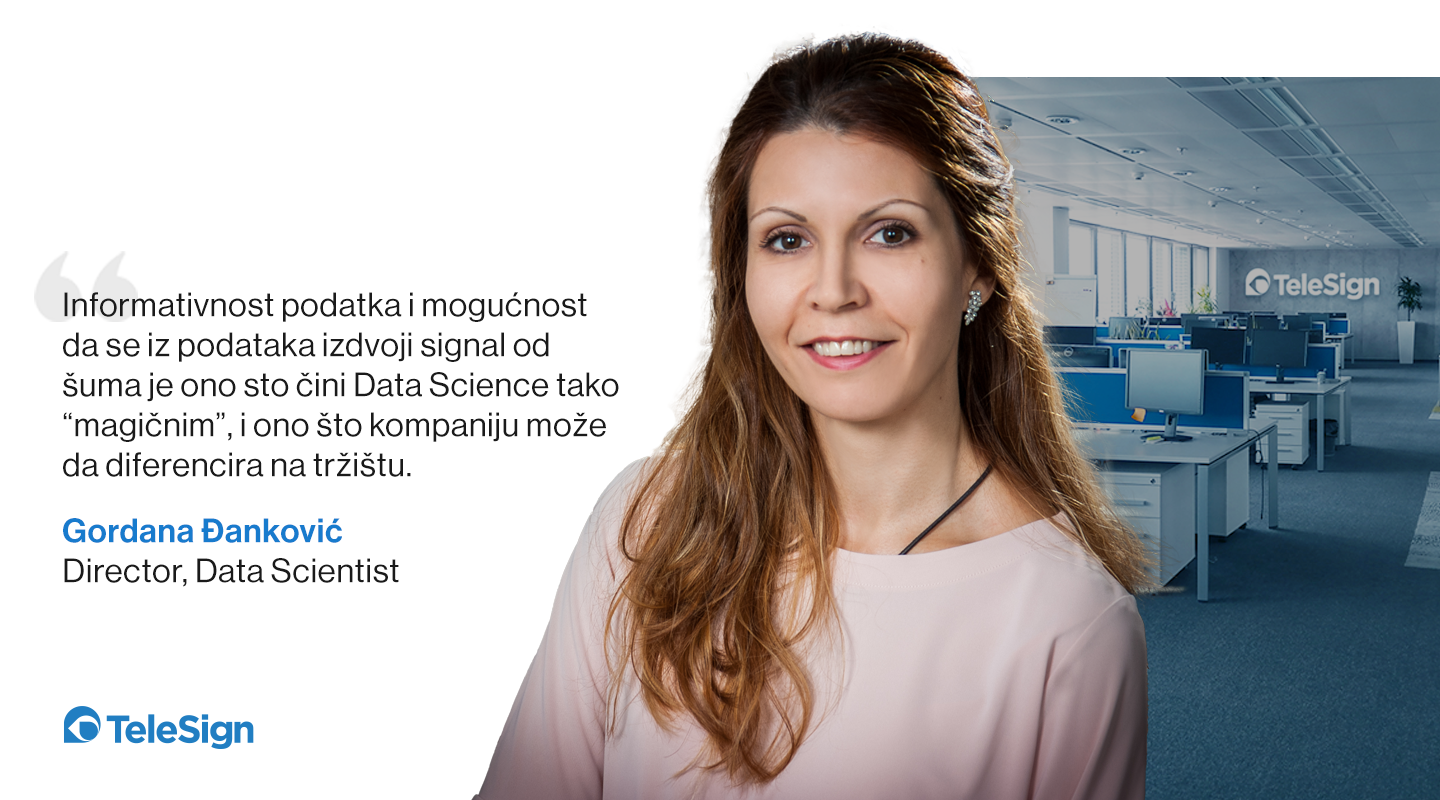
Do you need a Data Scientist, Data Engineer or Data Analyst?
We have already touched on the fact that someone needs to organize and maintain the data, that someone needs to find value in the data, and that someone needs to translate that value into long-term profit for the company. Is Data Scientist the best fit for all these roles? The answer to this question largely depends on the size of the company, the amount of available data and the level of maturity of the data-driven strategy, as well as the Data Science department within the company. For smaller companies, or those just starting their data journey, a Data Scientist, as someone who possesses analytical and research skills, as well as an enviable knowledge of Data Science technologies, is the best first choice. However, when the level is reached where Data Science in the company begins to grow from research into a potential product, the necessary profiles that will support research, technical and analytical Data Science work should be clearly defined - if we wanted to summarize those definitions in a few keywords, it would look like this:
1.Data Scientist - extractor of information from data, i.e. generator of valid business ideas,
2. Data Engineer – generator of an advanced data platform,
3. Data Analyst - connector in the relationship Data Engineer - Data Scientist - business.
At first sight of different responsibilities, these roles, together with quality data, form an unbreakable environment necessary for the successful transformation of Data Science concepts and ideas into business.
Data Science as a new wave of business
If we understand and adopt all these steps, we come to the conclusion that Data Science should at least significantly participate in business management if we want to create a profitable data-driven organization. Data Science should be done by those who direct the business with the innovation of their results, and who can describe the results, purpose and benefits of their work for the company and its users in a transparent business language. Therefore, if we succeed in understanding Data Science as a new wave of business led by technically savvy, mathematically educated, business-driven individuals, we can say that we have successfully implemented Data Science in business and that, with an acceptable dose of randomness, we are moving "in the right direction".
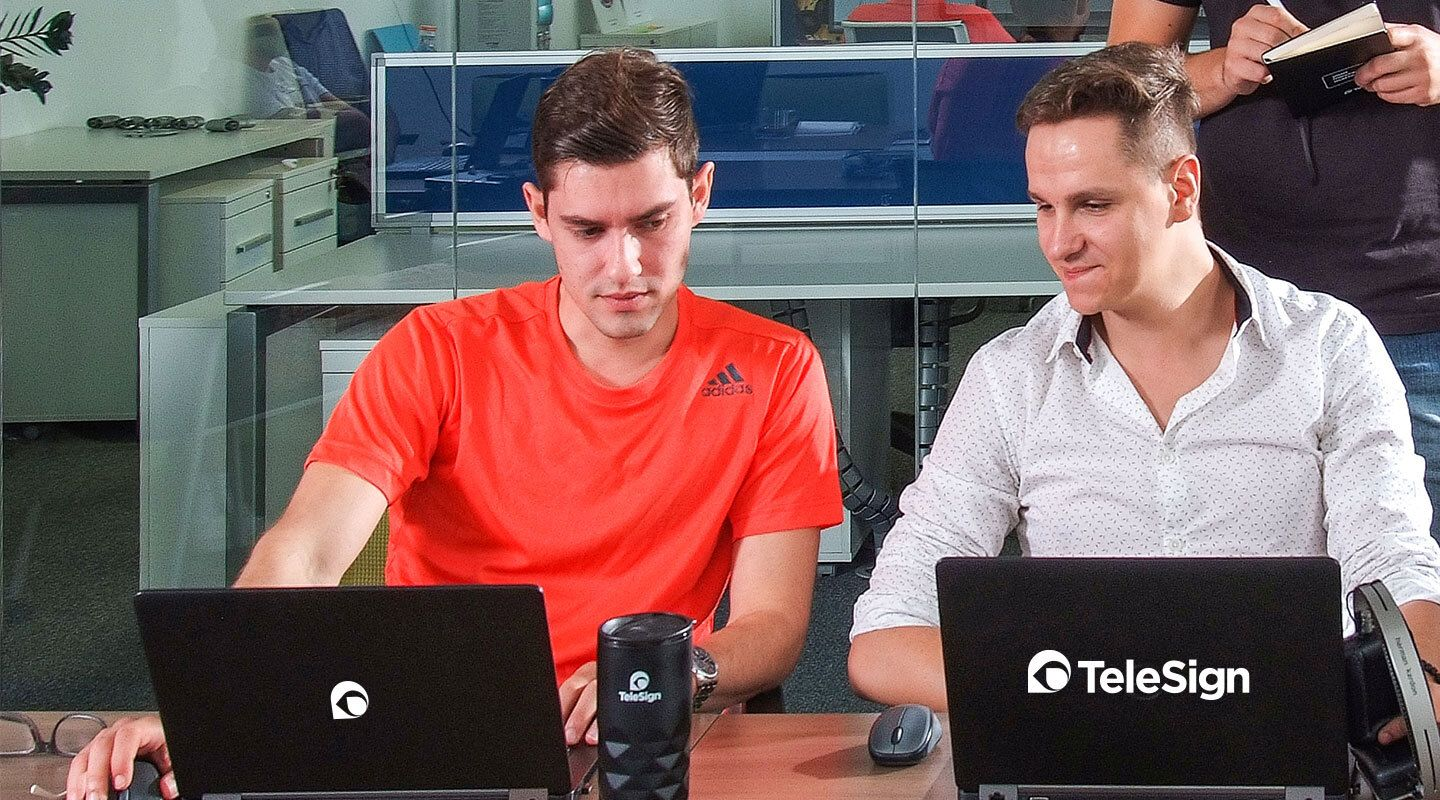